Every once in a great while, the question comes up: “How do I test my Databricks codebase?” It’s a fair question, and if you’re new to testing your code, it can seem a little overwhelming on the surface. However, I assure you the opposite is the case.
Building fun things is a real part of Data Engineering. Using your creative side when building a Lake House is possible, and using tools that are outside the normal box can sometimes be preferable. Checkout this video where I dive into how I build just such a Lake House using Modern Data Stack tools like AWS Lambda (for cheap and fast compute), DuckDB (for data processing) and Delta Lake for storage.
I’ve been playing around more and more lately with DuckDB. It’s a popular SQL-based tool that is lightweight and easy to use, probably one of the easiest tools to install and use. I mean, who doesn’t know how to pip install something and write SQL? Probably the very first thing you learn when cutting your teeth on programming when you’re wet behind the ears.
Well, another turkey day has come upon us all. I trust you are getting at least a day or two off from your overlords from writing code and taking names.
While the rest of you will be slicing up that turkey with your friends and family, clinking your glasses and giving toasts to each other, I will be stuck behind my glowing screen taping away at my keyboard producing juicy content for you all.
So, from now through this weekend, in a Black Friday sale extravaganza, %50 off memberships are available.
What do you get?
- Exclusive paid-only content (4-5 paid-only pieces per month)
- Full archives
- Data Engineering Central Podcast full access
- A hand in helping me bring the hammer down on unsuspecting SaaS victims.
I appreciate your support in helping me to continue to bring interesting and sometimes controversial content into the Data Engineering space.
We recently reached over 14k subscribers to the newsletter and about 17k followers on Substack.
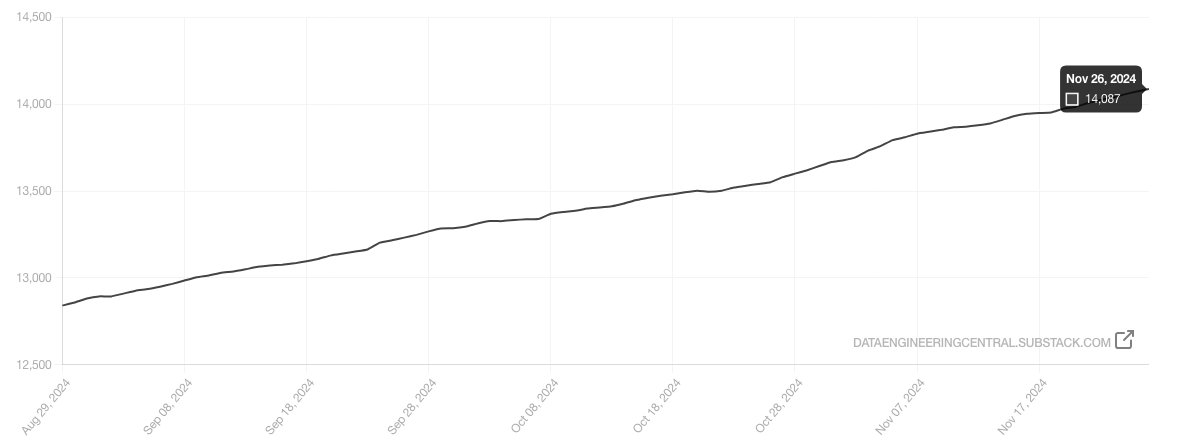
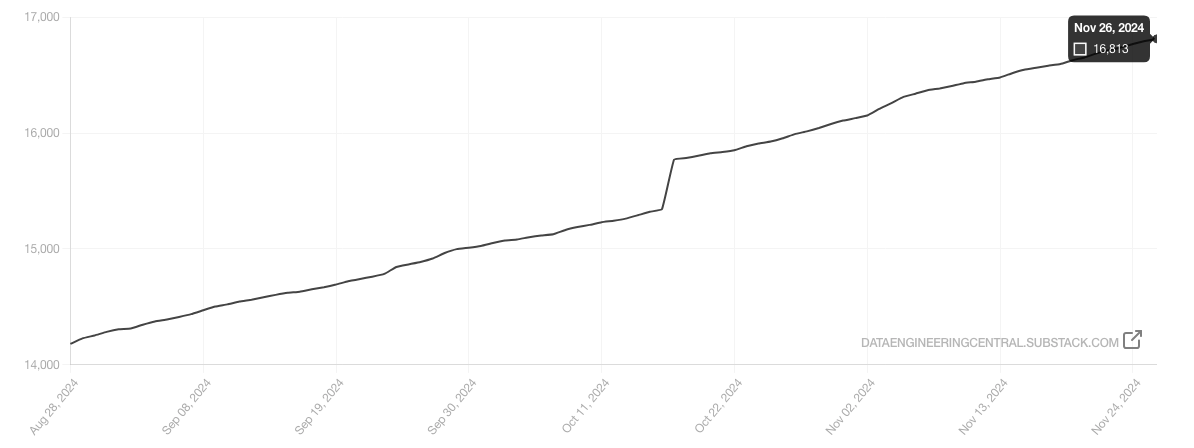
If you’ve ever been in the market for a Data Engineering job, or you’re alive and on Linkedin, you’ve probably been constantly inundated with job postings and requests pounding on your emails like a constant mountain stream even bubbling down a hill.
If that’s not the case, then head over to the quarterly salary discussion on r/dataengineering and cruise around the comments for an hour or two. One thing that will become clear quickly is that there is a huge range in pay scales, for apparently the same jobs.
One person is making 190K base and another one on the same stack is making 80K, it’s enough to make you pull your hair out. Heck, I get a constant stream of messages for “great opportunities” for a good 50K less than I make at this moment.
What’s the deal?
Sure, there is always going to be some disparity depending on the person who is the Data Engineer, some people simply deliver twice as much as others and are compensated accordingly. Some have over a decade of experience, some only a few.
Straight to the point.
I will tell you what the deal is. It’s the company(s).
The truth is that this sort of pay disparity exists all across the board, not only in Data Engineering, it’s a human phenomenon, and the Data field is no exception. Companies are simply different and act differently towards employees. Sometimes you’re a widget no one cares about, easily replaceable, and sometimes you end up in a place where people are core to what happens, and are paid accordingly.
- Companies that pay well below market value don’t care and probably have bad cultures.
- Companies that pay at or above market value are investing in people and have good cultures.
- Companies that don’t care about data don’t pay well and have crappy infrastructure and tooling.
- Companies that care about data pay well and have great infrastructure and tooling.
A lot of it boils down to two things.
- Does this company care about people generally, or not so much? Do they invest in people or see them as liabilities that are easily replaceable?
- Does this company care about “data” in a real way? Do they invest in their data?
If you’re a Data Engineer you should find a place to work that cares about people and data, the perfect cross-section. This will maximize your earnings. Although we should not complain as a whole as Data Engineers, salaries generally speaking on average or more than enough to live a good life on.
Overall, data engineers can expect to earn between $96,673 and $130,026 on average annually, with higher earnings possible in certain locations and for those with specialized skills and experience. – AI
How to make more money.
Do you want to make more money, are you underpaid? The answer is simple. Find a new job.
Humans don’t like change, many people get stuck and afraid to move on, and this is what keeps them operating in poor cultures where they are treated poorly, overworked, and underpaid. I’m here to tell you data is still the new oil, even more so with the rise of AI.
You can double you income simply by changing jobs every 1.5 years or so.
Nothing will give you a 20-30% pay bump quicker than changing jobs. You can work hard, even at a good company, and only make that sort of increase after working for a decade. Don’t do it.
Data Engineering pays well AT GOOD Companies. Go get paid.
I recently did a challenge. The results were clear. DuckDB CANNOT handle larger-than-memory datasets. OOM Errors. See link below for more details.
… DuckDB vs Polars – Thunderdome. 16GB on 4GB machine Challenge.
Are lambdas one of those tools that everyone uses and no one talks about? I guess I’ve taken them for granted over the years, even though they are incredibly useful. For a lot of my Data Engineering career I didn’t really think about or use AWS lambdas, I just saw them as little annoying flies on the wall, incapable of “real” use in Data Engineering pipelines.
But, I’ve changed my evil ways, and come to love the little buggers. Easy to use, cheap, and no infrastructure to worry about, I mean, they are little jewels in the rough.
I guess now that I look back and reflect, I’m surprised that I don’t see more content produced singing the praises and glories of AWS lambdas, why is the world silent? Those workhorses chug along in the background, millions and millions of times every second.
Today will not be earth-shattering, just a 10,000-foot view of AWS lambdas to inspire you to use them. With a focus on their use in Data Engineering.
Interesting links
Here are some interesting links for you! Enjoy your stay :)Pages
Categories
Archive
- March 2025
- February 2025
- January 2025
- December 2024
- November 2024
- October 2024
- September 2024
- August 2024
- July 2024
- June 2024
- May 2024
- April 2024
- March 2024
- February 2024
- January 2024
- December 2023
- November 2023
- October 2023
- September 2023
- August 2023
- July 2023
- June 2023
- May 2023
- April 2023
- March 2023
- February 2023
- January 2023
- December 2022
- November 2022
- October 2022
- September 2022
- August 2022
- July 2022
- June 2022
- May 2022
- April 2022
- March 2022
- February 2022
- January 2022
- December 2021
- November 2021
- October 2021
- September 2021
- August 2021
- July 2021
- June 2021
- May 2021
- April 2021
- March 2021
- February 2021
- January 2021
- December 2020
- November 2020
- October 2020
- September 2020
- August 2020
- July 2020
- June 2020
- May 2020
- April 2020
- March 2020
- January 2020
- December 2019
- November 2019
- October 2019
- September 2019
- August 2019
- July 2019
- May 2019
- March 2019
- February 2019
- January 2019
- December 2018
- November 2018
- October 2018
- September 2018
- July 2018
- June 2018
- May 2018
- April 2018
- March 2018
- February 2018