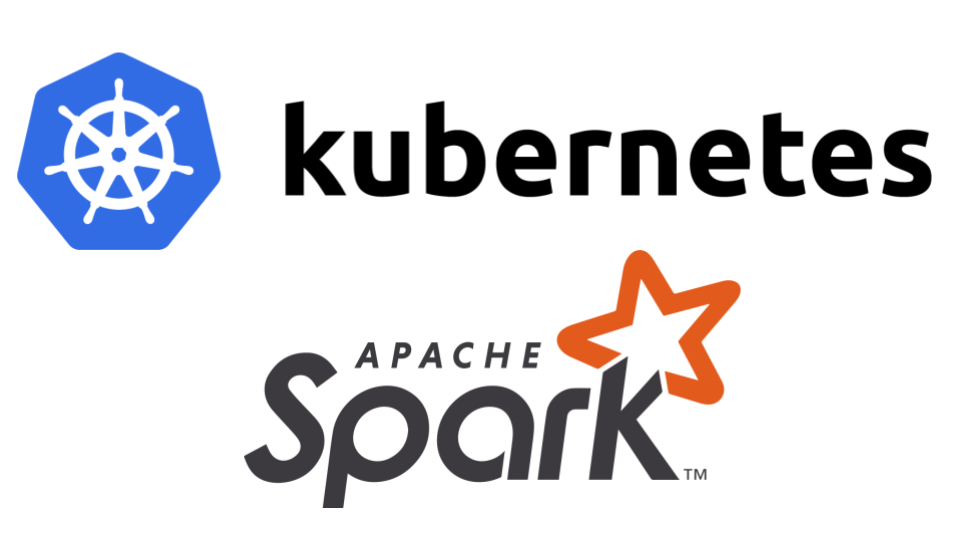
Data gets bigger and teams want to process data faster, what else can you do? There is only so much code tweaking you can do, threads, processes, asyncio, it’s only going to get you so far. At some point you have terabytes of data to process, and it requires a decision about some sort of distributed processing system.
In my experience I’ve mostly used two different distributed data processing systems in production, Spark and Kubernetes. To be honest the choice has always been obvious when to choose one over the other. The data usually dictates which system you choose. I’m sure there are super fans of each system who would argue there’s always a way to do any transform or process on each, but sometimes the point is, which system is setup to easily and quickly move the data from one point to another, and transform it as needed.
Read more