The blog post reviews an Apache Incubating project called Apache XTable, which aims to provide cross-format interoperability among Delta Lake, Apache Hudi, and Apache Iceberg. Below is a concise breakdown from some time I spend playing around this this new tool and some technical observations:
When it comes to building modern Lake House architecture, we often get stuck in the past, doing the same old things time after time. We are human; we are lemmings; it’s just the trap we fall into. Usually, that pit we fall into is called Spark. Now, don’t get me wrong; I love Spark. We couldn’t have what we have today in terms of Data Platforms if it wasn’t for Apache Spark.
This is an interesting one indeed, it’s one that teases and puzzles the brain to no end. Has the Data Warehouse finally died, has that unruly upstart the Lake House finally taken its place atop the seething mass of data we call home? Can we say that after all these decades the Data Warehouse Toolkit and Kimball is finally gone the way of the dinosaurs? Maybe. Probably. I don’t know.
It is a Brave New World out there these days. The new tools and features come out faster than your mom on Sunday morning getting you ready for church. The same goes for the context and advice being produced on a myriad of platforms, the ole’ Like and Subscribe, and all that bit. It does make you wonder after a while, what you can trust, who has your best interest in mind, and who is selling you a bottle of snake oil, doesn’t it?
Today we talk about Data Modeling. Specifically Data Modeling in the new world we all live in christened The Lakehouse by our benevolent Vender Overlords.
The battle for the Data Warehouse, Data Lake, Lake House, or whatever you want to call it, in the age of AI just got more interesting. In an unsurprising move, Databricks has announced plans to buy Tabular for 1 billion dollars, beating out Snowflake who was reportedly trying to do the same thing.
I still remember that day. A day that shall live on in infamy in my mind. Well over a decade ago, in the days when SQL Server roamed the land devouring souls on the Altar of Stored Procedures. There was only one tool available at the time. SQL. That’s it. There was one problem that had to be solved.
The answer? A recursive CTE.
At the same time … both a demon of the dark and a shining angel from the heavens. Just depends on your view.
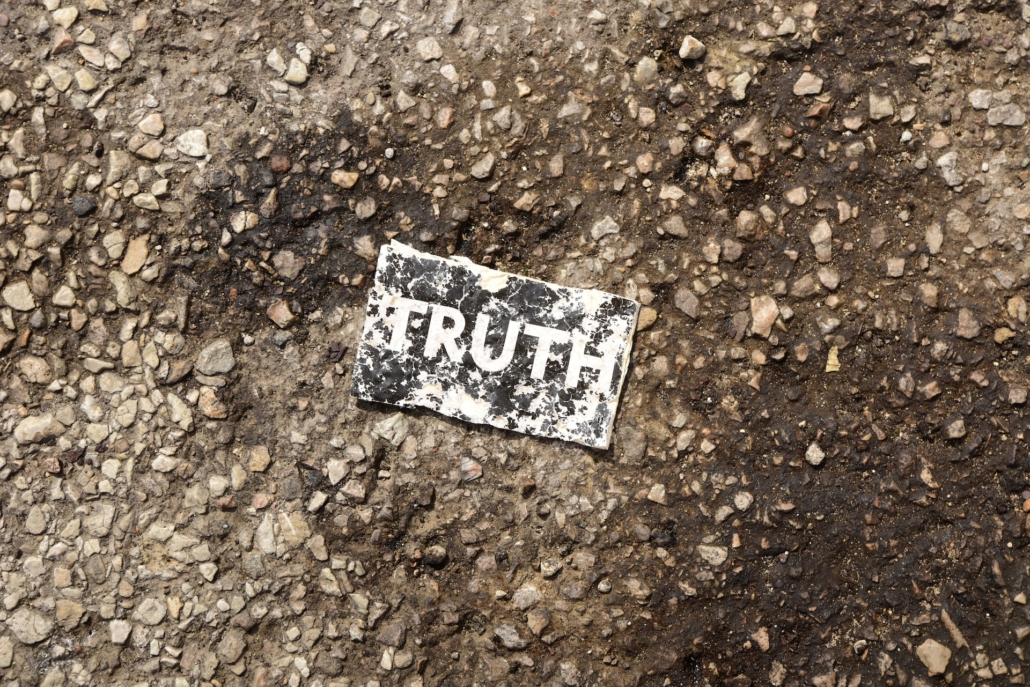
Photo by Michael Carruth on Unsplash
Anyone who’s been working in Data Land for any time at all, knows that the reality of life very rarely matches the glut of shiny snake oil we get sold on a daily basis. That’s just part of life. Every new tool, every single thingy-ma-bob we think is going to solve all our problems and send us happily into the state of nirvana inside our eternal data pipelines, is a lesson in disappointment.
I get it, there are a lot of nice tools out there. I use some of them every day. But, a healthy dose of reality is good for us all. Don’t lie to yourself. There is no such thing as the perfect tool. There are good tools, bad tools, and tools in between. The Truth is that all tools get pushed to their limits at some point.
We work on small teams, we don’t have all the time in the world, and we have to deliver our data at some point, perfect or not. We cut corners, hopefully, the right ones. That’s part of being wise and putting years of data experience to work. Today I’m going to talk about my experience of running Databricks + Delta Lake at scale. What happens when you use Databricks to ingest and deal with 10’s of millions of records a day, billions+ records a month?
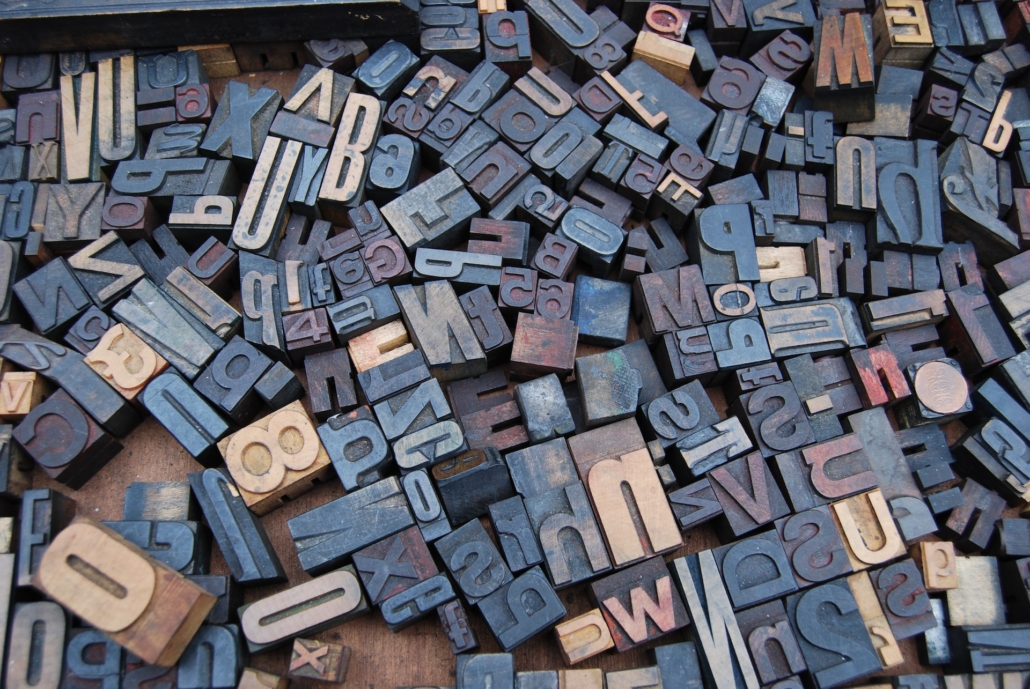
Photo by Amador Loureiro on Unsplash
Hmm … data types. We all know they are important, but we don’t take them very seriously. I mean we know the difference between boolean
, string
, and integers
, those are easy to get right. But we all get sloppy, sometimes we got the string
and varchar
route because we don’t spend enough time on the data model to care.
Can a string
versus a int
or bigint
in Delta Lake with Spark have a big impact on performance? Data size? Does it matter? Let’s find out.
Anyone who’s been roaming around the forest of Data Engineering has probably run into many of the newish tools that have been growing rapidly around the concepts of Data Warehouses, Data Lakes, and Lake Houses … the merging of the old relational database functionality with TB and PB level cloud-based file storage systems. Tools like Delta Lake, lakeFS, Hudi, and the like.
Sure, these tools have been around for some time, but the uptake and adoption of them all have been rapidly growing. I use Delta Lake on a daily basis, taking advantage of the many wonderful features it provides to simplify and reduce complexity in data pipelines. But, I’ve been sitting around for a long time waiting for the plethora of “add-on” tooling to come out, stuff that will make my life easier. I recently saw one of the first tools like that for Delta Lake, namely mack.
Mack appears to have the ability to “do the hard work for you,” a concept that appears to be growing in popularity, but which I have a fraught relationship with. Double-edged sword? Let’s find out.
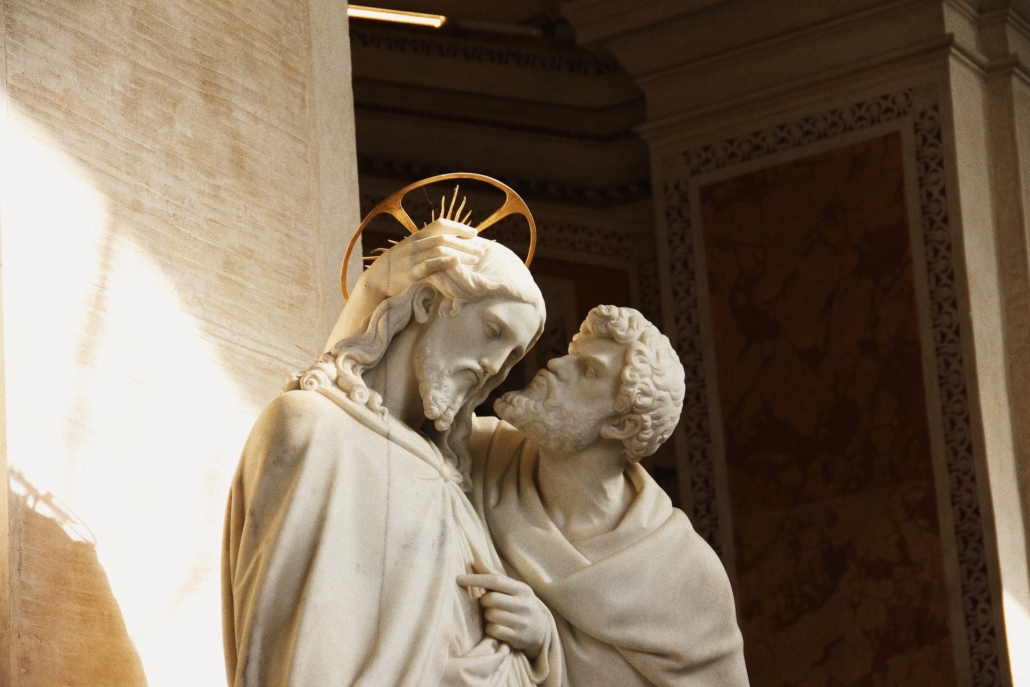
Nothing captures the imagination and heart like a tale of betrayal and heartbreak, and that is a tale I want to bring to you today. It’s a tale of Databricks Workflows and Jobs, version changes, new features, API’s, and insidious little hidden gems that will make you pull your hair out when you find them. It’s a tale of what not to do, a tale of how to put developer and customer experience first, instead of forcing unwanted solutions down the throats of the little birdies feeding at your nest.
As a Data Engineering simplicity and ease of use is something close to my heart, something that Databricks did well, or maybe I should say used to do well … before recent releases like Jobs 2.1 API.
I hope you can hear the bitterness oozing from my words.
Interesting links
Here are some interesting links for you! Enjoy your stay :)Pages
Categories
Archive
- March 2025
- February 2025
- January 2025
- December 2024
- November 2024
- October 2024
- September 2024
- August 2024
- July 2024
- June 2024
- May 2024
- April 2024
- March 2024
- February 2024
- January 2024
- December 2023
- November 2023
- October 2023
- September 2023
- August 2023
- July 2023
- June 2023
- May 2023
- April 2023
- March 2023
- February 2023
- January 2023
- December 2022
- November 2022
- October 2022
- September 2022
- August 2022
- July 2022
- June 2022
- May 2022
- April 2022
- March 2022
- February 2022
- January 2022
- December 2021
- November 2021
- October 2021
- September 2021
- August 2021
- July 2021
- June 2021
- May 2021
- April 2021
- March 2021
- February 2021
- January 2021
- December 2020
- November 2020
- October 2020
- September 2020
- August 2020
- July 2020
- June 2020
- May 2020
- April 2020
- March 2020
- January 2020
- December 2019
- November 2019
- October 2019
- September 2019
- August 2019
- July 2019
- May 2019
- March 2019
- February 2019
- January 2019
- December 2018
- November 2018
- October 2018
- September 2018
- July 2018
- June 2018
- May 2018
- April 2018
- March 2018
- February 2018