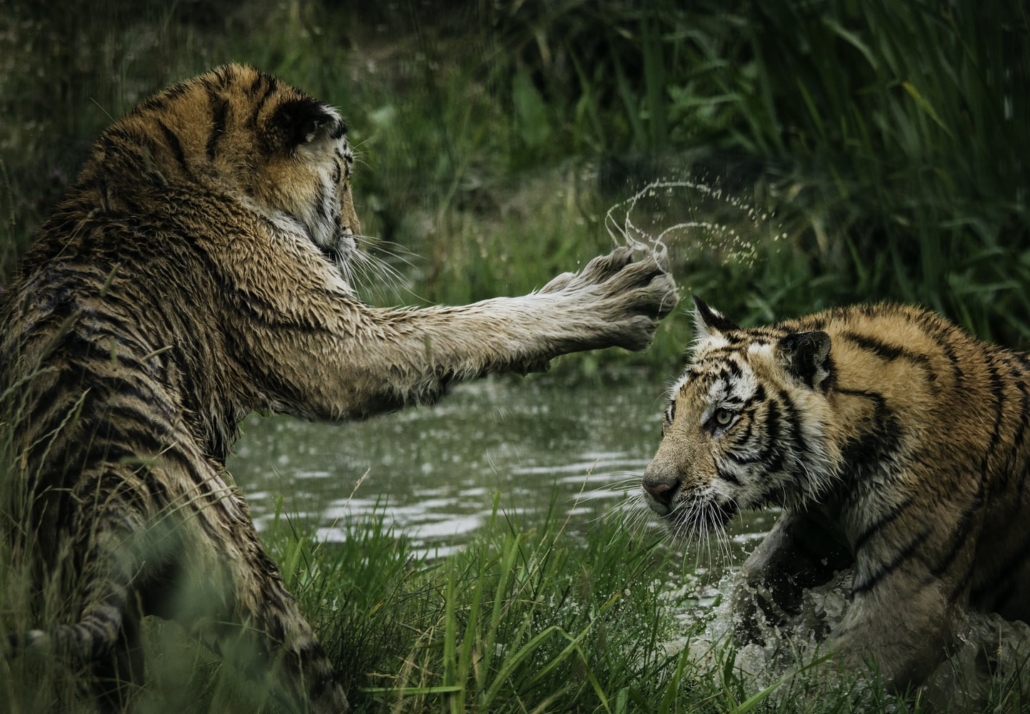
Dagster, the first few times I read the name, I just couldn’t take the tech stack seriously …. it’s still kinda hard. Today I want to compare Airflow vs Dagster, mostly explore what Dagster is and does. But I want to compare it to the popular Apache Airflow project so people have some context for it. It’s kinda hard keeping up on all the new stuff these days, I usually just wait till I see enough articles and tweet floating around about it, then I know it’s maybe worth a peak. Let’s crack open Dagster, and see if it’s better then the name chosen for it.
Read more