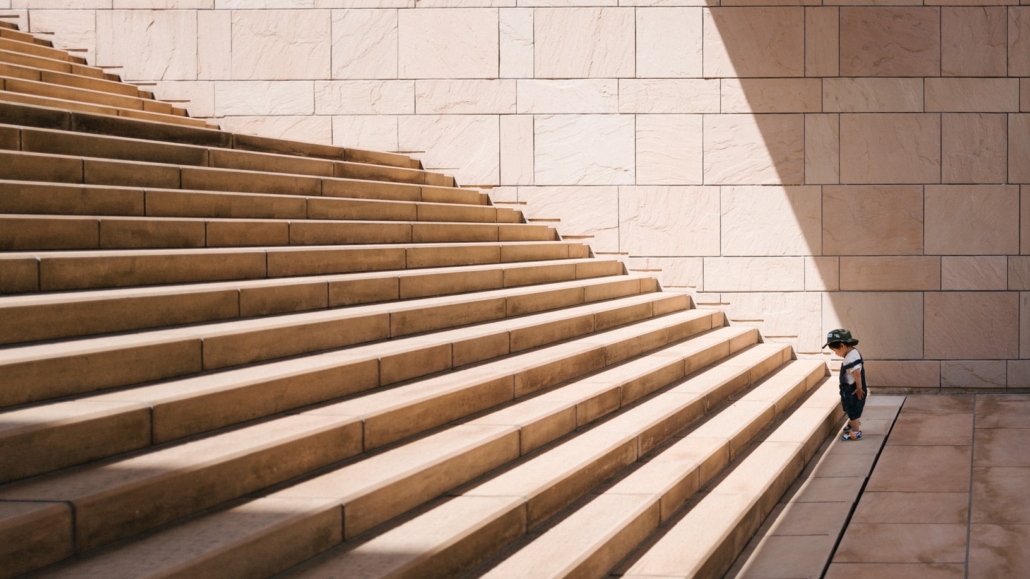
It’s not often I yearn for the good old days of SQL Server
, but I’ve had a few of those moments lately. Some things I miss, some I don’t, and it’s probably because I’m getting old and crusty, stuck in my ways, by permissioning
is one of those topics where I think about the good old days. Data access control and permissions are topics that we all kinda ignore as not that important … until we actually are trying to do something with them. Then all of sudden we start complaining about complexity and why this isn’t easier. That’s a good way to describe my reaction to having to work on Databricks Access control.
But, I learned a few things and I think they will be helpful for someone. Read on for the basics of handling permissions and access control in Databricks and Delta Lake.
Read more