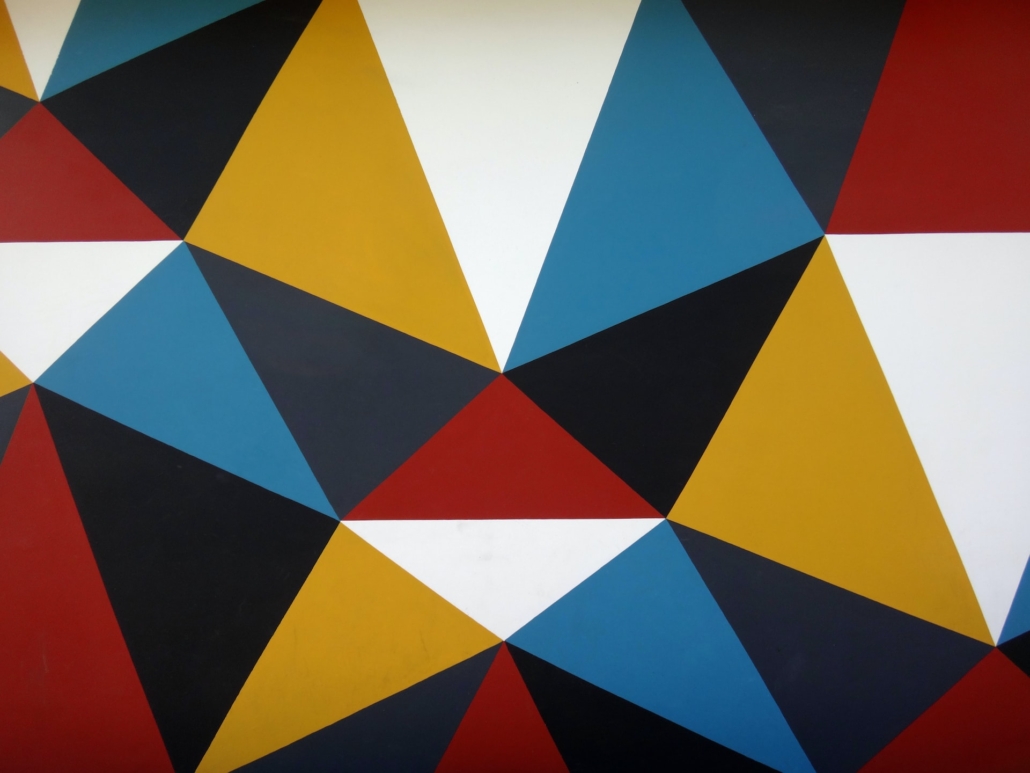
When I think back many moons ago, to when I started in Data Engineering world … even though it went by many different names back in the olden days … I didn’t know what I didn’t know. All those years ago Kimball’s Data Warehouse Toolkit was probably the only resource really available at the time that touched on the general concepts that most “Data Engineers” at the time were working on. The field has come a long way since those days and changed for the better, it’s less often you see classic Data Warehouses running on legacy SQL Servers, with stored procedures with hundreds and thousands of lines of SQL code.
That had me thinking about designing data load patterns in the Modern Data Stack. I want to talk about general data loading patterns, how to design your data pipelines, at a high level, and the basic principles and practices that apply to 99% of all the transformations and data loads done by most Data Engineers.
Read more